Data Scientist - a.k.a. Data Science specialist, or who? In short, a Data Scientist is a person who, among other things, collects, processes, analyzes and visualizes data using machine learning and learning algorithms. Data Scientist is, in very simple terms, a combination of the professions of Data Engineer and Data Analyst. A Data Science specialist must have skills and competencies from many different fields. He or she should be able to program, be familiar with big data technologies and data analysis and additionally have communication skills and understand the business. Why? We explain it in detail in the article below.
Who exactly is a Data Scientist?
Is Data Scientist the latest buzzword, or is there actually some new meaning behind the term? We hear from many sources that this is the profession of the future, the most in-demand role in IT, and at the same time an interesting career path, full of challenges and interesting problems to solve.
We have grown accustomed to the demand for statisticians, data analysis and machine learning specialists, as well as programmers. The trend of recent years, however, has been the convergence of these previously separate roles. In the context ofbig data processing, it is now difficult to deal with machine learning, for example, in isolation from performance issues and planning for production deployment of developed solutions. Business expects real value, in the form of convincingly communicated conclusions and predictions or production-performing solutions, not hermetic analyses understood only by domain experts.
In view of the above, it seems that the term Data Scientist has succeeded in highlighting the expectation of combining in a single role the qualities that until now have been dispersed among specialists in different areas, rather than identifying some entirely new role.
Is this expectation realistic to fulfill? Can one person actually be both a great programmer, a statistician dissecting algorithms on a cluster, a person with a thorough understanding of the business within which the company operates, and at the same time have high communication skills and be able to communicate their conclusions and predictions in the form of beautiful infographics and charts?
This is undoubtedly a big challenge for recruiting departments, hence the many semi-ironic comparisons of the search for Data Scientists to the search for a unicorn. It can be assumed that the profile described above to a certain unmatched benchmark, the ideal employee of a modern company, who is able to transcend the boundaries of the usual departments within the company and use deep subject matter knowledge to transform the data available to the company into a real benefit.
In certain situations, this kind of specialist becomes a project manager who, even if he is not directly involved in such different fields of activity, has to manage a team and cooperate with the many parties involved. He then becomes a liaison between data analysis experts and developers, and domain experts and management.
Data Scientist in Polish - translation problems
With the term Data Scientist there is a problem in Polish - how to translate it? Unfortunately, it is impossible to literal, data analyst does not reflect the full meaning of the role, the data master promoted by Wikipedia sounds quite strange and foreign, some try with data scientist. In this version, in turn, we lose the connection with the name of the field - Data Science - which in the original clearly suggests that we are even dealing with a separate discipline. In general, the field of data analysis and machine learning is unlucky when it comes to terminology in Polish, e.g. slick data mining can't be pronounced equally fluently in Polish as data digging, the most sensible being the slightly distant data mining. So, for lack of better options, let's stay with the original Data Scientist.
An attempt to discuss the issue of translating Data Science in Polish was made by Norbert Ryciak in the article: Danetics, or about the Polish translation of Data Science
Data Scientist skills and requirements
When working in Data Science, it is important to have numerous competencies from many different specialties. It is desirable on the job market that a Data Scientist should have mathematical and analytical skills, be able to program, be able to present analyzed data and draw specific conclusions. In addition, such a person should be characterized by curiosity, the ability to tell stories through data (data storytelling) and understand the needs of the business. This is shown in detail in the graphic below.
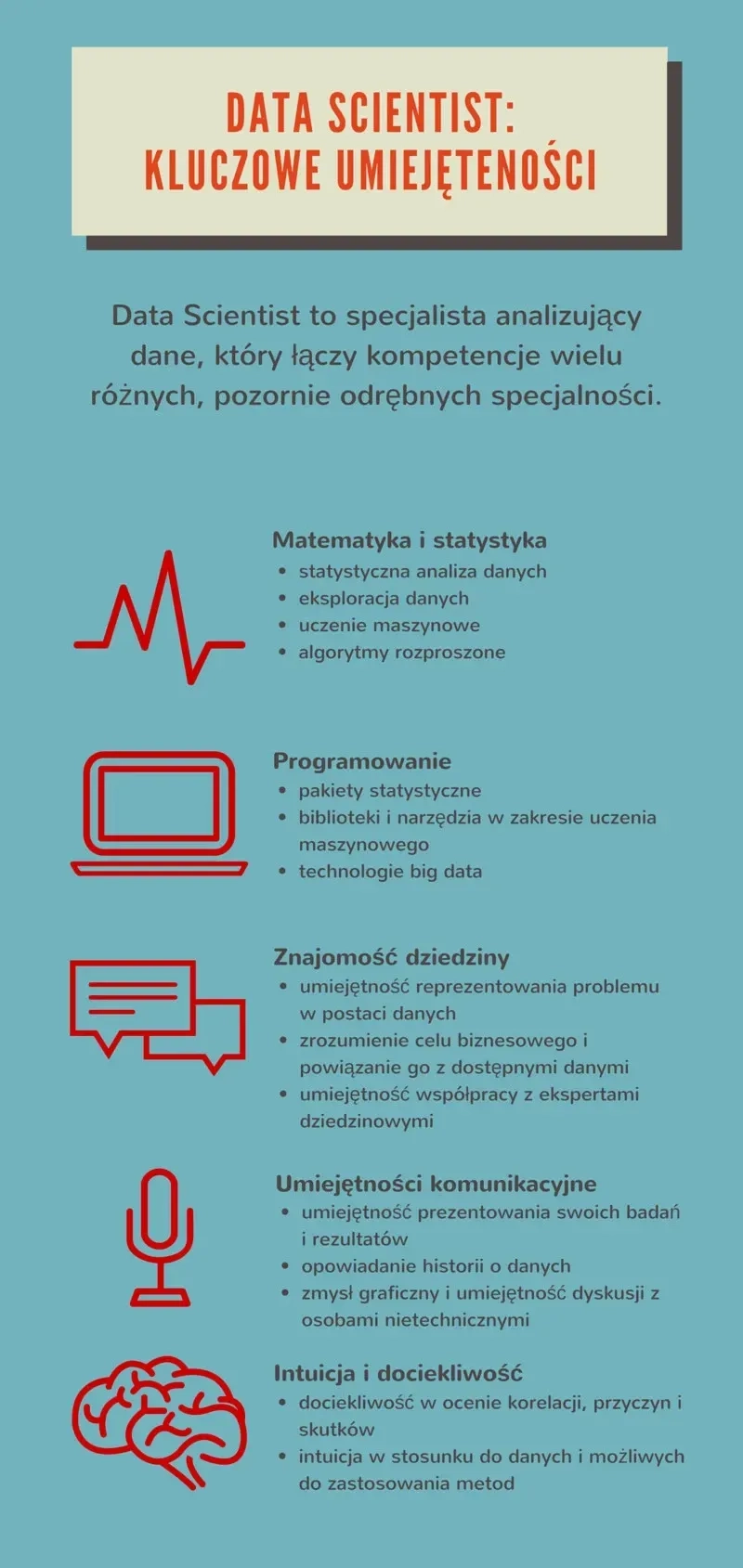
Data Scientist: desirable qualities. Are we looking for a unicorn?
Why is everyone looking for Data Scientists right now?
The question arises why just now --- in the last few years --- has there been this kind of shift in relation to expectations of employees? First, it is the aforementioned impact of the big data trend and the need to deal with such large volumes of data that implementation and technical issues become important early on in data processing.
Secondly, it is also the realization by companies that in virtually every business, collecting, analyzing and processing data carries potentially high value. The more companies use this kind of activity to increase their competitive advantage, the more it fuels the overall race in which no one wants to be left in the tail.
Third, data processing is becoming increasingly accessible, both because of the plethora of platforms, tools and libraries for this purpose, as well as some developments in the field of machine learning itself. We're talking about deeplearning of neural networks*(deep learning*), a group of methods that have made it so that suddenly in many applications, results previously achieved by laborious experimental work (matching methods and features to a given problem) are now possible virtually out of the box.
Let's look at the search trends below. Machine learning (green) is a buzzword that was rather losing popularity by 2011. This is a period of disillusionment with the fact that, despite many years of development in this field, we are still not living in a world out of science fiction books, light years away from true artificial intelligence, and the systems currently in place are quickly proving to be very limited or even useless, contrary to marketing promises.
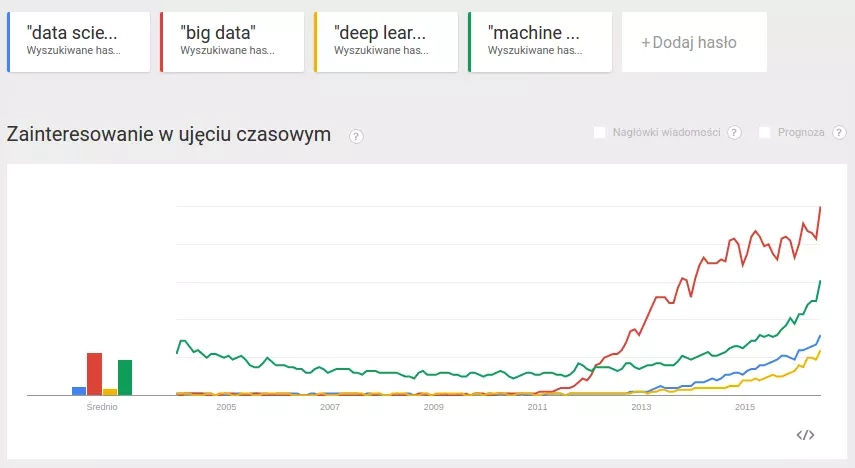
Search trends for the keyword Data Scientist and related
The year 2011 also marked the beginning of the hype of the term big data, a splash of the idea that it is possible and worthwhile to collect all the data at hand, because once it is fed into the right computing machine, we will gain crucial knowledge that was previously inaccessible to us. The period of disillusionment with this concept began quite recently.
An interesting correlation can be observed between the popularity of the slogan "deep learning" (yellow) and "data science" (blue). The temporal coincidence seems to support the hypothesis that the applicability of this group of machine learning methods reinforces the trend of searching for people who have competence in this field and at the same time know how to use it to deliver value to the company.
What do Data Scientists do?
At this point it would probably be appropriate to ask: what areas don't Data Scientists work in yet? We actually deal with data analysis in every field and industry, one can only say where it is crucial or most promising.
In the financial industry, it is crucial to analyze data on banking transactions and support credit decisions, such asfraud detection, which makes it possible to identify the most suspicious operations and pass them on to a human for further analysis.
In marketing, analyzing user behavior on websites, such as online stores, is proving very valuable. This makes it possible, among other things, to create more and more perfect recommendation systems, indicating to the customer products that he is more likely to buy, even if he is not actively looking for them.
Another interesting area of the Data Scientist's work is tracking brand visibility and opinion on the Internet through the use of natural language processing solutions. Particularly for global brands, it is important to monitor and respond to changing perceptions of a company expressed by statements on the Internet, such as in product reviews or simply in social network discussions. Without automated analysis, this would be downright impossible, due to the volume of data or limited to small samples. Using automated overtone analysis and big data solutions, all key areas of the Internet can be monitored.
For almost any business, it is extremely important to analyze sales data --- predicting sales trends, or segmenting customers. This allows you to make decisions that affect the company's strategy in terms of product offerings, or the functioning of the sales department.
An extremely interesting trend in recent years is the concept of open data: making data available in a structured form, from public institutions, administration and other sources relevant to citizens, and their subsequent analysis. The first step here often proves to be the most difficult and crucial, as breaking down administrative barriers is akin to fighting windmills, but getting the data out into the public domain offers a chance for any interested citizen to potentially turn the released information into real value for others. An example of this is the analysis of the causes and possible solutions to the formation of traffic jams in a city, based on spatial data about the speed of cars on the roads, at particular hours of the day.
How to become a Data Scientist?
Until recently, specialists were divided into those who gained their competence at universities and those who apprenticed themselves while working. Nowadays, we are seeing completely new trends in education, drawing attention to the advantage of alternative ways of acquiring knowledge over higher education, and the need to constantly update one's knowledge, throughout life*(lifelong learning*).
A manifestation of the first of these trends are bootcamps, intensive courses focused on imparting participants with very practical knowledge, enabling them to start working in their profession, such as the Data Science bootcamp at Kodołamacz.pl. In the second case, online education is now playing an increasingly important role, particularly MOOCs*(Massive Open Online Courses*), which allow anyone to access university-level knowledge remotely via a web browser.
Such innovative approaches to education have their advantages and disadvantages, of course; in particular, bootcamps are not a direct substitute for several years of study at the master's or even engineering level. They allow you to quickly gain basic practical competencies so that you can get to work and further develop your skills while already performing everyday tasks. Online courses, on the other hand, are an excellent learning resource, often at the level of the world's best universities, but they can't replace face-to-face contact with a teacher and the motivation provided by being in a physical group of students.
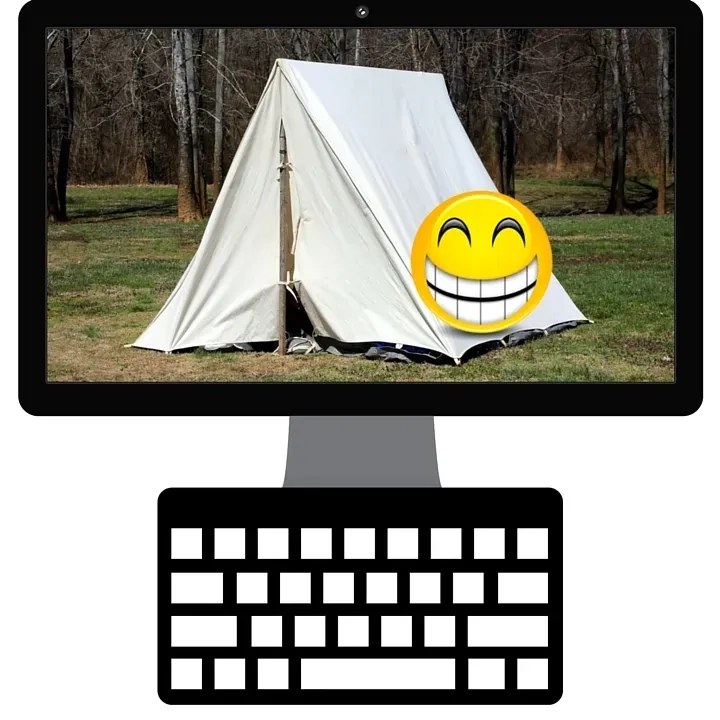
Bootcamps --- are they fulfilling their role?
The field of Data Science is particularly problematic here, since the competence base of a person in this role is a strong mathematical and statistical foundation. This kind of knowledge is difficult to assimilate in a course of several weeks. It is not out of the question, of course, but it still requires a long tenure in the workplace to gain the necessary experience. It seems, therefore, that the classical model of university education is still leading the way here.
Complementing the offer of master's degrees, whose curriculum is often basic and does not cover specialized issues, and often fails to keep up with rapidly changing technologies, are postgraduate studies. On the Polish market, several universities offer Data Science studies, in particular, this academic year at the Warsaw University of Technology two postgraduate tracks will be open: Data Science and Big Data (disclaimer: Sages and the author are directly involved in the organization of the aforementioned postgraduate studies and bootcamps Kodołamacz.pl).
If you want to start a career in this industry, be sure to read Michał Kardasz's article: How to find your first job in Data Science?
Regardless of your educational background, the issue of lifelong learning is exceptionally relevant in the context of Data Science, as it encompasses the most dynamically changing areas of today's reality, such as programming technologies, or data analysis and processing. This area is helped by materials available online (MOOCs), courses and training, or membership in thematic interest groups and meetups.
How do you build your career as a Data Scientist?
Finally, a few words about what you can do while already being a Data Scientist to enhance your image in the market and develop your career in a thoughtful way. The Kaggle service comes to mind, which has now become a modern and dynamic resume for Data Scientists, much like GitHub for programmers.
First of all, the Kaggle service is a platform that hosts contests for the best solutions to problems, related to data analysis, announced by companies from all over the world. The competitions are open to anyone who proposes an algorithmic solution to a given problem based on the description and sample data provided. In this way, he builds his "resume", which is a showcase of the person, supported by actual empirical data on the effectiveness of the proposed methods. On the other hand, Kaggle is a platform for finding top talent: companies interested in recruiting can search the database of said electronic resumes and select the person with the most suitable profile.
Like programmers, many data analytics professionals function on portals like StackExchange, assisting others with answers to questions. In addition, of course, there are the classic ways of documenting and describing one's work, such as conference and academic journal publications.
What else? Let us know in the comments.