I am a business analyst. I help clients implement changes that add value to their business. I assist in identifying the right solutions - by "right," I mean those that deliver real benefits and are tailored to the client's context.
In this post, I would like to share some thoughts on the role of Artificial Intelligence (AI) and Data Science (DS) in the work of a business analyst. This is one of the "hot" topics today, so it's worth delving into.
Artificial Intelligence vs. Data Science
As an introduction, many people confuse the concepts of artificial intelligence and Data Science. For some, they are the same, while others believe that artificial intelligence is a broader topic than DS. In reality, the relationship between these areas is a bit more complex. DS serves as the foundation for AI, but the field itself extends far beyond AI. This is illustrated by a popular graphic found online:
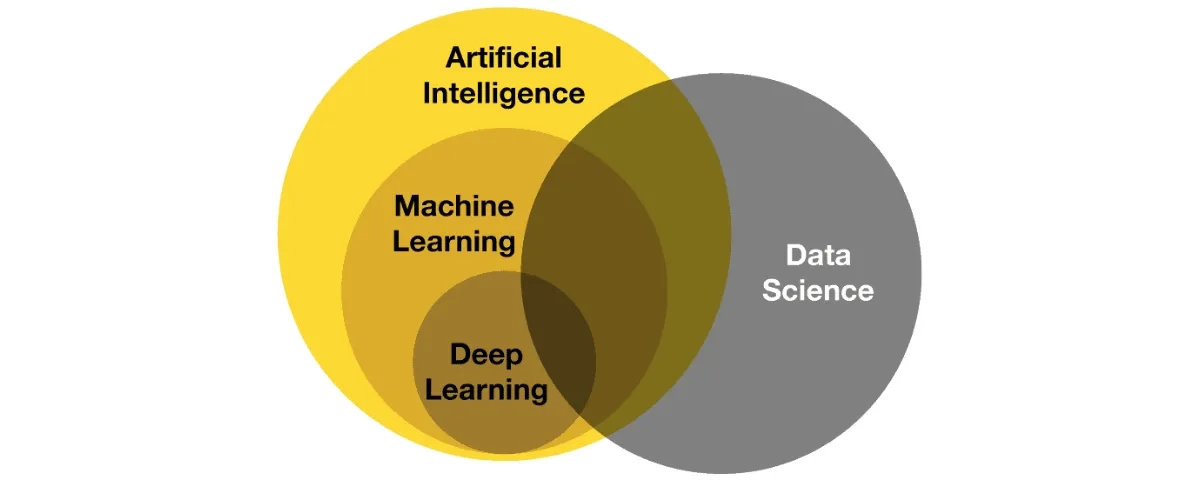
Artificial Intelligence (AI) - is a broad concept encompassing the development of mechanisms capable of simulating human thinking, reasoning, and behavior. Machine Learning (ML) - is a subset of AI in which computer systems learn from their environment and use that knowledge to improve experiences and processes. All machine learning technologies are fundamental to AI, but not all AI relies on machine learning. Deep Learning (DL) - is a part of the broader family of machine learning methods based on artificial neural networks. DL uses multiple layers to progressively extract higher-level features from raw input. Data Science - involves processing, analyzing, and drawing meaningful insights from data. In simpler terms, it's about discovering hidden patterns in data. Data scientists use machine learning to predict future events.
Application of DS and AI in Business Analysis
Do Data Science and artificial intelligence have applications in the work of a business analyst? Absolutely. Both areas can help in several specific areas of an analyst's work: strategic analysis, solution development, requirements engineering.
Supporting Strategic Analysis
In strategic analysis, Data Science and AI can help identify new business development directions through data analysis and the identification of important patterns. For example, we can use data analysis to determine new business needs based on insights extracted from the data. An example of this is analyzing historical sales data, combining it with factors that may affect sales, such as market trends, political conditions, or economic factors. Then, based on the analysis of historical data and influencing factors, we can make predictions about future sales. This allows decision-makers to make decisions regarding, for example, launching a new product offering, not based on intuition or gut feeling, but on data.
Another example of using Data Science in strategic analysis is process improvement. Imagine the following situation: we gather information on who and how currently performs business processes in the organization. Data analysis can identify areas of activities in the process that are not being carried out according to procedures or are not being carried out at all. Based on this analysis, an algorithm can suggest the need for improvement or updating of these process areas. It may also turn out that specific actions in the process are unnecessary, and users have found a "shortcut." In such a case, it may be that certain steps in the process are simply unnecessary.
Performing such analysis without the use of algorithms and data would be very difficult because it would likely require observing users, taking notes, or recording user actions, comparing observations with process documentation, and drawing conclusions through manual or partially manual processes. Data Science and artificial intelligence can greatly simplify this task for us. However, this does not mean that artificial intelligence will make the decision that a certain step in the process is unnecessary. The final decision is a business decision and should be left to the domain expert. Artificial intelligence can facilitate this decision-making process by presenting properly processed data. That's where the power of tool support lies.
AI and Data Science also excel in automating certain tasks, such as in the insurance sector. An algorithm can analyze data to detect fraud and pass potential cases to be verified by an insurance company employee. This way, we relieve people from the need to perform manual data analysis - instead, they can focus on more strategic activities and aspects that bring greater business value.
Data Science allows us to harness the power of data, which is still a challenge in many companies. Data is available in the organization, but we do not use it to support business decisions. Perhaps this is because data exists but is scattered and difficult to interpret, or it exists but there are no efficient tools for its analysis. As a result, many decisions made by management turn out to be incorrect because they are based not on facts but on guesswork or intuition. It is important to remember that the quality of business decisions largely depends on the quality of data. Therefore, it is worth relying on facts and data to minimize the risk of failure and achieve better business results.
In summary, at the strategic level, data analysis and artificial intelligence can help domain experts and business professionals make informed decisions about development strategies, identify new business needs, and introduce new solutions. However, it is important to understand that artificial intelligence can only assist in presenting processed data and pointing out certain patterns. The final decision on business significance should be left to decision-makers because algorithms operate only based on what they have learned and the quality of the data they have learned from. At this stage of technology development, algorithms do not invent solutions on their own, do not predict many events, and do not have "business intuition." Therefore, caution should be exercised when using artificial intelligence because its effectiveness depends on the quality of data and cannot replace human decision-making and intuition in key business matters.
Supporting Business Initiatives
Another example of using Data Science and artificial intelligence is supporting business initiatives such as marketing strategies and specific promotional actions. We understand that when introducing a new solution (product, service), it is important to take care of marketing and advertising. Otherwise, our excellent product or service may simply go unnoticed in the market, and potential customers may not be aware of the new offering. While marketing is not a typical area of interest for "project" analysts dealing with system solutions, business analysts operating in the strategic domain should also consider sales and marketing aspects.
In this context, generative artificial intelligence can help us generate specific marketing strategies and content for posts and publications, significantly reducing the time required for these tasks. Although artificial intelligence can be very helpful, it should not replace human thinking and verification. It is crucial to exercise caution and verify the quality of generated content to avoid publishing incorrect or misleading information.
Without sufficient knowledge to check the generated text, there is a risk that the content will be factually incorrect and mislead the audience. From a business perspective, publishing a message that misleads the audience can have serious negative consequences. For example, a message published on social media, generated by artificial intelligence and not verified by individuals with the relevant knowledge, may create expectations among the audience that are impossible to fulfill. Blindly following technology and believing in its infallibility is a risky move that can lead to negative consequences for a company's reputation and customer relationships. Therefore, we treat artificial intelligence support as assistance, and we should not accept information provided by AI as absolute truth.
In my opinion, artificial intelligence is a tool that supports certain activities but is not (yet) a replacement for human competence and experience. It is valuable to treat AI as support, a tool - and verify the results provided by this tool. Business decisions should be made by people with the relevant knowledge and experience in the specific field. I see enormous potential for the evolution of business analysis as a discipline. In the future, many of our competencies may focus on providing the right data and content to AI algorithms to enable drawing conclusions based on properly prepared data. This will allow us to save time and dispense with some of the tasks currently performed, such as gathering information on a specific topic or conducting market research. This data can be acquired or presented automatically by systems. As a result, we will be able to focus on the proper interpretation of this data and drawing conclusions. We will be able to propose new solutions, business offers, and identify areas for improvement in a more efficient way, based on a wider range of data. This undoubtedly promises an exciting future for business analysis.
Supporting Solution Concept Development
How else can artificial intelligence help us? It can support the selection or development of the right solutions for business problems by suggesting solution recommendations for similar challenges. With data collected within the organization and publicly available, Data Science and artificial intelligence have incredible potential. We no longer have to rely solely on our knowledge, perspective, and experience to propose the right solution for a business problem. Thanks to generative artificial intelligence, we can learn about solutions to other similar business problems available on the market, although, of course, with certain limitations. This opens up new possibilities and enables more accurate decision-making.
Solutions based on artificial intelligence, such as the popular chatGPT, may seem tempting due to potential time and effort savings, but it is important to exercise caution, especially regarding information security. Providing confidential company data to chatGPT or similar tools to obtain development strategy insights may carry the risk of breaching confidentiality and data security.
Customer and company data security should be our priority. Let's not forget this while being fascinated by technological innovations. Artificial intelligence technologies have their limitations and do not replace thorough analysis and decision-making by people. Let's use these tools as support for the thought process while ensuring the security and trust of our customers. This way, we can reap the benefits of new technologies while protecting the interests of our clients.
How else can artificial intelligence support the work of a business analyst? It can provide suggestions for solving business problems, suggest specific functions or requirements suitable for a given context, and even expedite the content generation process or quality control of artifacts. For some time now, attempts have been made to generate diagrams based on text. In my opinion, these attempts have been rather unsuccessful due to the poor quality of the provided texts and algorithm limitations, but we can hope that the results will become more useful over time. It is essential to note one simple fact - results largely depend on the quality of the provided data. Artificial intelligence relies on algorithms that learn from data. If the data and patterns are incorrect, good results cannot be expected. This is a principle that applies to virtually all systems engineering. If we start with a low-quality product, the outcome or result will likely not meet high standards.
Conclusion
In conclusion, AI and Data Science are incredibly valuable tools for business analysts, supporting us in making more accurate, informed decisions, automating tasks, and discovering hidden patterns. With their help, we can add more value to the business and achieve better results in our analytical work. However, like any tool, it is essential to use common sense and focus on defining the purpose and potential application of the tool rather than using the tool itself as the goal.
Special thanks to Ross Apostoł, who introduced me to the world of AI.